Inter-observer and intra-observer reliability between manual segmentation and semi-automated segmentation for carotid vessel wall volume measurements on three-dimensional ultrasonography
Article information
Abstract
Purpose
Carotid vessel wall volume (VWV) measurement on three-dimensional ultrasonography (3DUS) outperforms conventional two-dimensional ultrasonography for carotid atherosclerosis evaluation. Although time-saving semi-automated algorithms have been introduced, their clinical availability remains limited due to a lack of validation, particularly an extensive reliability analysis. This study compared inter-observer and intra-observer reliability between manual segmentation and semi-automated segmentation for carotid VWV measurements on 3DUS.
Methods
Thirty-one 3DUS volume datasets were prospectively acquired from 20 healthy subjects, aged >18 years, without previous stroke, transient ischemic attack, or cardiovascular disease. Five observers segmented all volume datasets both manually and semi-automatically. The process was repeated five times. Reliability was expressed by the intraclass correlation coefficient, supplemented by the coefficient of variation.
Results
Carotid VWV measurements using the common carotid artery (CCA) were more reliable than those using the internal carotid artery (ICA) or external carotid artery (ECA) for both manual and semiautomated segmentation (manual segmentation, CCA: inter-observer, 0.935; intra-observer, 0.934 to 0.966; ICA: inter-observer, 0.784; intra-observer, 0.756 to 0.878; ECA: inter-observer, 0.732; intraobserver, 0.919 to 0.962; semi-automated segmentation, CCA: inter-observer, 0.986; intra-observer, 0.954 to 0.993; ICA: inter-observer, 0.977; intra-observer, 0.958 to 0.978; ECA: inter-observer, 0.966; intra-observer, 0.884 to 0.937). Total carotid VWV measurements by manual (inter-observer, 0.922; intra-observer, 0.927 to 0.961) and semi-automated segmentation (inter-observer, 0.987; intra-observer, 0.968 to 0.989) were highly reliable. Semi-automated segmentation showed higher reliability than manual segmentation for both individual and total carotid VWV measurements.
Conclusion
3DUS carotid VWV measurements of the CCA are more reliable than measurements of the ICA and ECA. Total carotid VWV measurements are highly reliable. Semi-automated segmentation has higher reliability than manual segmentation.
Introduction
The carotid artery is a common site for atherosclerosis [1-3]. Carotid atherosclerosis is primarily associated with gradual vessel wall remodeling, as well as atherosclerotic plaque formation. Plaques with a high level of vulnerability may rupture and possibly embolize cerebral arteries, leading to ischemic stroke. In particular, thrombo-embolism constitutes a large proportion of reported ischemic stroke cases [4]. Longitudinal screening for plaques or tracking their progression can be effective for estimating the risk of rupture and predicting potential stroke in asymptomatic patients [5-8]. As a non-invasive, radiation-free, and widely accessible imaging modality, two-dimensional ultrasonography (2DUS) is conventionally applied for routine monitoring. Carotid intima-media thickness (CIMT) [9] and blood flow velocity [10,11] are well-established 2DUS metrics. However, CIMT is relatively non-specific, as other factors can concurrently contribute to focal intimal thickening, such as hypertensive medial hypertrophy [12,13] or mechanical forces from normal blood circulation [14,15]. Blood flow velocity is also limited as an indirect indicator of the spatial distribution underlying stenosis severity [16].
In recent research, three-dimensional ultrasonography (3DUS) has emerged as a method of illustrating the anatomical and functional characteristics of the arterial wall in a fully volumetric manner [17-19]. Beyond outperforming 2DUS, 3DUS offers morphological complexity, vulnerability, and composition information for evaluating carotid atherosclerosis [20]. The total plaque volume (TPV) [21,22] and carotid vessel wall volume (VWV) [22,23] are the 3DUS phenotypes for such quantification. However, measuring the TPV requires a high degree of expertise to discern the plaqueouter vessel wall and plaque-lumen boundaries, hindering the measurement efficiency [21,22]. Carotid VWV, meanwhile, is considered more practical for clinical application. The measurement only demands a simpler interpretation of some regular, low-ordered, and circular vessel boundaries [22,23]. The carotid VWV is defined as the volume between the media-adventitia boundary and the lumen-intima boundary of the carotid artery. Since fatty deposits, scar tissues, and macrophages accumulate in the sub-intima [24-26], incorporating the subintimal layer in carotid VWV measurement enables quantifying the plaque burden. In practical terms, carotid VWV can be measured by two systematic approaches: total carotid VWV and individual carotid VWV. Total carotid VWV generally refers to the VWV summation of any two or more carotid branches [6,21,23]. In the present study, total carotid VWV was defined as the VWV summation of all three carotid branches: the common carotid artery (CCA), internal carotid artery (ICA), and external carotid artery (ECA). This single value can thereby summarize the overall carotid plaque burden within a patient. Individual carotid VWV is another measurement approach that quantifies the VWV of CCA, ICA, and ECA independently. In contexts such as the longitudinal monitoring of plaque progression and regression, the individual carotid VWV approach is more cost-effective for assessing the carotid branch of interest, rather than all three branches, in regular follow-up examinations.
Despite its significance, carotid VWV assessment has not been commonly applied in current clinical practice. Tedious manual segmentation and inadequate research on validation have been the major obstacles [27]. Even though numerous studies have accordingly introduced semi-automated segmentation algorithms to minimize computation and operation time [6,23,27-30], these segmentation methods have yet to be verified; in particular, a comprehensive reliability analysis is lacking. It is important to establish reliability measures when serial carotid VWV is being tentatively considered as a way to surveil temporal changes in arteries and plaques. While these algorithms allow a reduced user-interaction time, a comparison between methods of segmentation is also crucial to explore any potential trade-offs and detriments to consistency. The previous literature has mainly discussed the reliability of total carotid VWV, showing high inter-observer and intra-observer reliability for both manual and semi-automated segmentation [6,21,23]. However, the reliability of individual carotid VWV has not been thoroughly documented with either manual or semi-automated segmentation. The key uniqueness of this study is its extensive analysis of regional differences in the reliability of carotid arteries for VWV measurement with respect to manual and semi-automated segmentation. Since Egger and his team [21] validated VWV as a 3DUS imaging phenotype of carotid atherosclerosis, Hossain et al. [6] and Khan et al. [23] have only compared the reliability of manual segmentation and semi-automated segmentation for total carotid VWV measurements. In this context, the present study aimed to evaluate and compare the inter-observer and intra-observer reliability between manual segmentation and semi-automated segmentation in 3DUS for the CCA, ICA, and ECA individually, as well as total carotid VWV measurements.
Materials and Methods
Compliance with Ethical Standards
The study was approved by the Institutional Review Board of the authors’ institution (HSEARS20210908003). All participants provided written informed consent to the protocol before the commencement of the study.
Study Population
Power analysis using PASS software version 21.0.2 (PASS, NCSS, East Kaysville, UT, USA) showed that at least 29 3DUS volume datasets of the carotid artery were required. To account for possible drop-outs, the study conservatively recruited 20 subjects to measure their left and right carotid arteries in order to fulfill the required number of datasets.
Overall, a total of 20 healthy participants were enrolled in this study. The subject inclusion criteria were >18 years old, without previous stroke, transient ischemic attack and cardiovascular disease. Given that the existing literature has advocated using carotid VWV for asymptomatic plaque screening and future stroke prediction [5-8], the present study targeted a population with no previous history of atherosclerosis. The exclusion criterion was the presence of any 3DUS volume dataset with obscured carotid arteries by participants’ anatomical or postoperative factors (e.g., mandibular obscuration by a highly situated carotid bifurcation, mandibular obscuration by arthritis-induced limited head rotation, or obscuration by surgical clips, surgical sutures, central lines, or postoperative bandages) [31]. Participants were publicly recruited via the researchers’ social media platforms. Demographic information was documented prospectively along with comorbidities, such as obesity, diabetes mellitus, coronary artery disease, smoking, and hypertension.
3DUS Volume Acquisition
All ultrasound scanning procedures were performed on both the left and right carotid arteries of each participant, by using a Samsung RS80A ultrasound machine (Samsung Medison Co., Ltd., Seoul, Korea) in conjunction with a 3-14 MHz volume ultrasound transducer (LV3-14A, Samsung Medison Co., Ltd.). For each 3DUS carotid volume acquisition, one observer was randomly drawn from five observers (with 4 years of experience in radiography) to conduct the scanning procedures independently. The carotid arteries of the 20 subjects were allocated to five observers to be scanned; hence, there was only one observer per carotid artery in 3DUS volume acquisition. All observers received the same 3DUS training and had similar scanning experience. They were deemed to demonstrate equivalent abilities through a variability trial prior to scanning the subjects’ carotid arteries.
Upon participant registration, an initial 2DUS scan under grayscale and color Doppler ultrasonography was then undertaken with the volume transducer to verify the anatomical location of the CCA, ICA, and ECA. The carotid bifurcation was also centered in accordance with the 2DUS imaging.
Followed by the 3DUS volume acquisition, a commercially available S-3D Arterial Analysis (Samsung Medison Co., Ltd.) inbuilt software was used to reconstruct and display the volume. The volume acquisition axis was adjusted parallel to the longitudinal axis of the CCA. The matrix fanning angle was set as high as possible (30°) to maximize visualization of the vessel’s longitudinal portion. Technical parameters, such as focal zone, depth, brightness gain, and time-gain compensation, were optimized according to the individual carotid volume. The acquisition was initiated with a constant perpendicular insonation angle and no cardiac triggering. The transducer was held steadily during data capture. Participants were simultaneously instructed to arrest their breathing to reduce respiratory motion artifacts.
The acquired 2D image slices were automatically rendered into a 3D volume dataset using the software. All image slices were examined by an expert ultrasonographer (with more than 7 years of experience in ultrasonography) to ensure an acceptable image quality for carotid VWV evaluation. In this study, nine out of 40 3DUS volume datasets were excluded due to the inability to visualize the ICA and ECA because of subjects’ highly situated carotid bifurcation. The data exclusions were confirmed as a failure to obtain an appropriate image by an expert ultrasonographer, with all observers’ approval. For the remaining 31 volume datasets, the volumes were stored in the ultrasound unit for subsequent image analysis and segmentation.
Carotid VWV Segmentation
The same five observers conducted segmentation. All observers were trained and assumed to demonstrate equivalent abilities in carotid VWV segmentation. A variability trial was also conducted before the analysis of participant data. Both manual segmentation and semi-automated segmentation were repeated five times in a 24-hour interval by each of the observers. Overall, 1,550 segmentations were conducted in the study (31 volume datasets×2 types of segmentation×5 repetitions per observer×5 observers). The 3DUS volume datasets were re-randomized for every segmentation session. The observers were blinded to subject information and identification to suppress rater bias.
The volume datasets were segmented in the commercial S-3D Arterial Analysis inbuilt software (Samsung Medison Co., Ltd.) to compute carotid VWV. Under a 2D transverse view in the 3D volume, the media-adventitia boundary and lumen-intima boundary were traced slice by slice with the use of a mouse-driven cross-hair cursor. The interslice distance was pre-set as the lowest possible level (0.5 mm) to enhance segmentation accuracy on the boundaries [27]. In order to be compatible with other preliminary research on carotid VWV segmentation reliability, this present study employed a consistent methodology in terms of segmenting the same number of transverse slices for each subject volume [21,22]. As such, the length of interest for CCA segmentation covered 7.5 mm proximal to the carotid bifurcation, while the ICA and ECA segmentation both spanned 5 mm distal to the carotid bifurcation (Fig. 1).
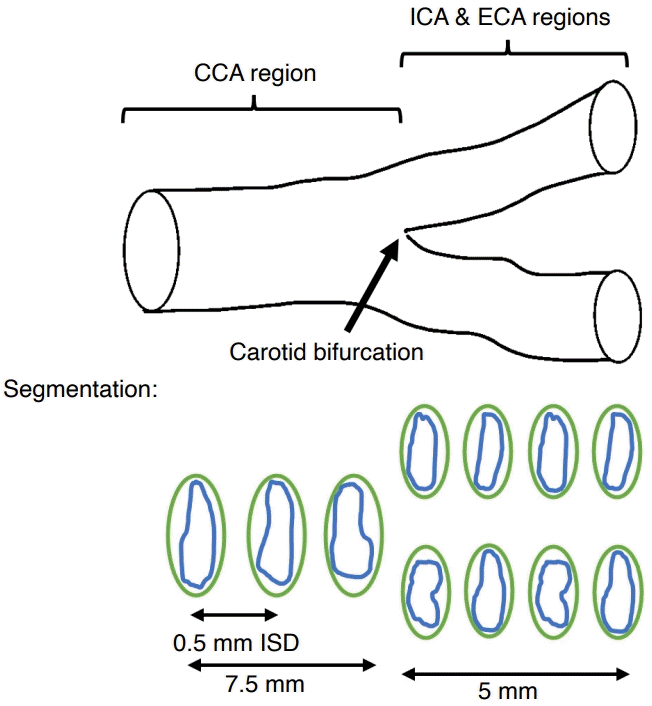
Schematic diagram showing the technical parameters of segmentation in carotid vessel wall volume measurement.
The media-adventitia boundary (green lines) and lumen-intima boundary (blue lines) were segmented under an interslice distance of 0.5 mm, with the length of interest being 7.5 mm proximal to the carotid bifurcation for CCA and 5 mm distal to the carotid bifurcation for both ICA and ECA. CCA, common carotid artery; ICA, internal carotid artery; ECA, external carotid artery; ISD, interslice distance.
In manual segmentation, observers delineated the media-adventitia boundary and lumen-intima boundary sequentially on every 2D transverse slice, to form a series of media-adventitia contours and lumen-intima contours, respectively. In semi-automated segmentation, observers randomly selected and annotated one reference 2D transverse slice, followed by an automatic segmentation in the software on the remaining frames. Once the contours were outlined by either segmentation method, the software processed each transverse slice by subtracting the area enclosed by the two contours from one another. Carotid VWV was then iterated by interpolating the subtracted area successively along the longitudinal length of interest by trapezoidal rule. Carotid VWV values were output and recorded as cubic millimeters. Fig. 2 shows an example of transverse slices with contoured media-adventitia and lumen-intima boundaries of the carotid arteries; Fig. 3 demonstrates the associated process flow of generating carotid VWV after contouring.
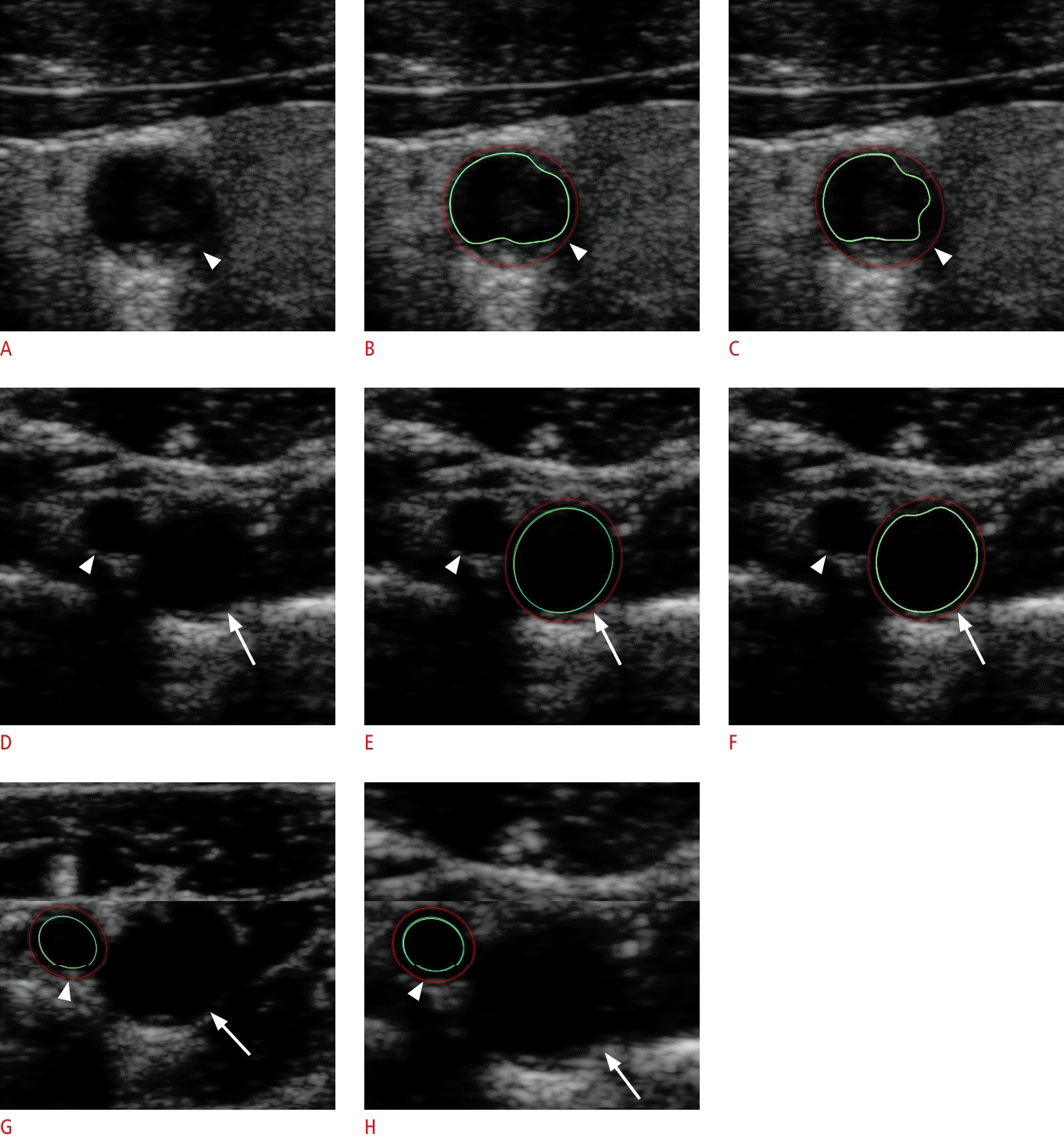
A 48-year-old healthy woman without previous stroke, transient ischemic attack and cardiovascular disease.
The media-adventitia boundary (red lines) and lumen-intima boundary (green lines) were contoured for the corresponding vessel of interest in the transverse slices of the three-dimensional ultrasound volume. A-C. The left common carotid artery (arrowhead) without segmentation (A), with manual segmentation (B), and with semiautomated segmentation (C) are shown. D-H. An unsegmented left internal carotid artery (arrow) and an unsegmented left external carotid artery (arrowhead) are shown (D). The left internal carotid artery (arrow) with manual segmentation (E) and semi-automated segmentation (F), and the left external carotid artery (arrowhead) with manual segmentation (G) and semiautomated segmentation (H) are also shown correspondingly.
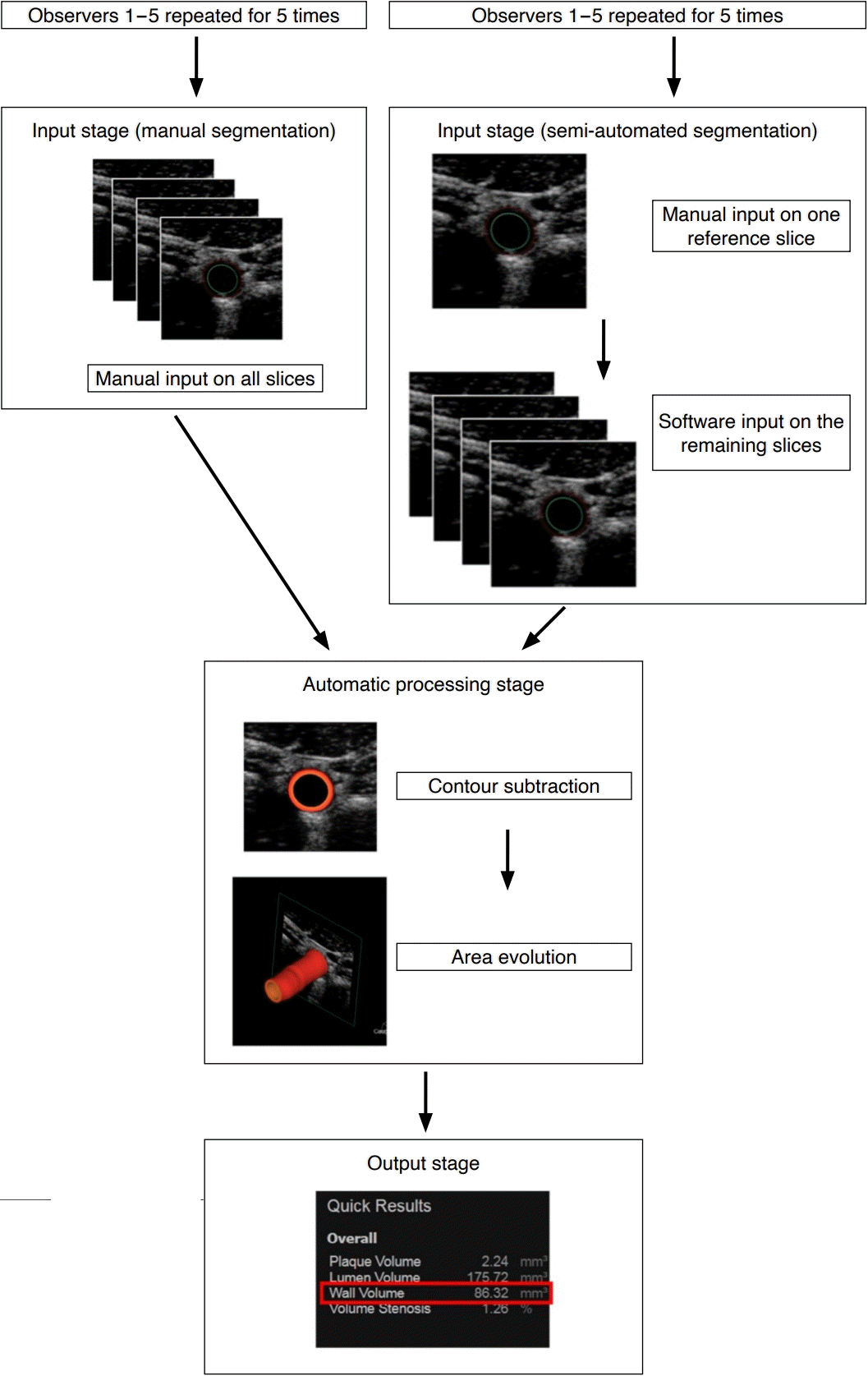
Flow diagram of generating carotid vessel wall volume by manual or semi-automated segmentation on three-dimensional ultrasonography.
The input stage involved contouring the media-adventitia boundary and lumen-intima boundary on two-dimensional transverse slices manually or semi-automatically, depending on the approach under investigation. In the automatic processing stage, the areas enclosed by the two contours were subtracted from one another in each transverse slice; and the subtracted area was incrementally evolved across the interslice distance using the trapezoidal rule. In the output stage, carotid vessel wall volume values (red box) were generated. The entire process was repeated until all five observers performed manual segmentation and semi-automated segmentation five times each.
The procedures were repeated across manual segmentation and semi-automated segmentation for the CCA, ICA, and ECA. The total carotid VWV was retrospectively derived by summing the three carotid VWVs of the individual carotid arteries.
Data Analysis
SPSS version 27.0 (IBM Corp., Armonk, NY, USA) was used to determine inferential statistics. The accuracy of the segmentation data was preliminarily evaluated. In this study, manual segmentation results were considered the ground truth of carotid VWV measurements [6,21,23]. Bland-Altman plots and Pearson correlation coefficients were applied to explore the respective agreement and correlation between manual segmentation and semi-automated segmentation for carotid VWV. The significance criterion was set at 0.05.
Inter-observer and intra-observer reliability values were expressed as the intraclass correlation coefficient (ICC), with the 95% confidence interval (CI) for a supplementary comparison. For inter-observer reliability, a mean value of VWV was obtained by averaging the measurements of the five segmentation repetitions of each observer. The SPSS input was tabulated as 31 subject volumes against five observers. As a mean rating was considered, an ICC (2, k) model in two-way random-effects analysis of variance (ANOVA) was applied to analyze inter-observer reliability [32]. For intra-observer reliability, the individual VWV value measured by each observer was assessed under a single rating approach. The SPSS input was tabulated as 31 subject volumes against five time-point measurements. An ICC (3, 1) model in two-way mixed-effects ANOVA was conducted for intra-observer reliability analysis [32].
For descriptive statistics, the coefficient of variation (CoV) was used to complementarily depict relative variance. the CoV was calculated as the percentage of standard deviation relative to the mean.
Results
From November 2021 to January 2022, a total of 20 healthy participants (6 men and 14 women; mean age, 30.7±14.7 years; age range, 21 to 60 years) were enrolled in the study. Demographic information and neurovascular risk factors are listed in Table 1. In brief, all participants had no known neurovascular risk factors associated with carotid atherosclerosis.
In this study, nine out of the 40 3DUS volume datasets were excluded because it was not possible to visualize the ICA and ECA due to participants’ highly situated carotid bifurcation. Finally, 31 3DUS volume datasets were included in the study. A total of 1,550 sets of segmentation were generated by five observers with five repetitions. Table 2 summarizes the measured carotid VWV and stenosis percentages by 3DUS in this study. Considering measurements from manual segmentation in relation to baseline demographics, the mean total carotid VWV of the recruited participants was 154.03±19.63 mm3; while the mean carotid VWV of CCA, ICA, and ECA were 73.79±11.48 mm3, 46.86±7.33 mm3, and 33.39±5.84 mm3, respectively.
The Bland-Altman plots shown in Fig. 4 reflect the agreement of the segmentation data by comparing semi-automated segmentation against manual segmentation. The results confirm that the mean difference of the semi-automated segmentation mostly fell within±1.96 standard deviation of manual segmentation for all measurements (CCA, ICA, ECA, and total carotid VWV). The mean bias values of the respective carotid VWV measurements (CCA VWV, -21.57 mm3; ICA VWV, -15.33 mm3; ECA VWV, -4.64 mm3; and total carotid VWV, -41.54 mm3) also remained a small proportion (ranging between 12.2% to 24.6%) relative to the mean carotid VWV values obtained from the 31 volume datasets in this study. The Pearson correlation coefficients between manual and semi-automated segmentation were 0.465 (P<0.001) for CCA VWV, 0.481 (P<0.001) for ICA VWV, 0.663 (P<0.001) for ECA VWV, and 0.601 (P<0.001) for total carotid VWV measurements. Therefore, manual segmentation and semi-automated segmentation were both accurate, as demonstrated by both agreement and correlation, for generating the carotid VWV. The established accuracy also allowed a further analysis of carotid VWV measurements, particularly in terms of reliability, as the main study findings.

Bland-Altman plots for agreement between manual segmentation and semi-automated segmentation for carotid vessel wall volume (VWV) in the common carotid artery (A), internal carotid artery (B), external carotid artery (C), and total carotid VWV (D).
SD, standard deviation.
The inter-observer reliability between manual segmentation and semi-automated segmentation for carotid VWV measurements on 3DUS is shown in Table 3. Regarding the carotid VWV measurements of the individual carotid arteries, manual segmentation of the ICA (ICC, 0.784; 95% CI, 0.420 to 0.910; CoV, 15.57%) and ECA (ICC, 0.732; 95% CI, 0.437 to 0.872; CoV, 16.80%) yielded lower inter-observer reliability than that of the CCA (ICC, 0.935; 95% CI, 0.709 to 0.977; CoV, 8.50%). Semi-automated segmentation of the ICA (ICC, 0.977; 95% CI, 0.906 to 0.991; CoV, 9.01%) and ECA (ICC, 0.966; 95% CI, 0.803 to 0.989; CoV, 10.65%) similarly showed lower inter-observer reliability than that of the CCA (ICC, 0.986; 95% CI, 0.927 to 0.995; CoV, 7.17%). For the total carotid VWV measurements, semi-automated segmentation, with an ICC value of 0.987 (95% CI, 0.930 to 0.996; CoV, 5.48%) attained higher inter-observer reliability than manual segmentation (ICC, 0.922; 95% CI, 0.750 to 0.969; CoV, 6.82%). Semi-automated segmentation also yielded higher ICC values than manual segmentation for the individual carotid arteries, with reference to the above-reported inter-observer reliability values.
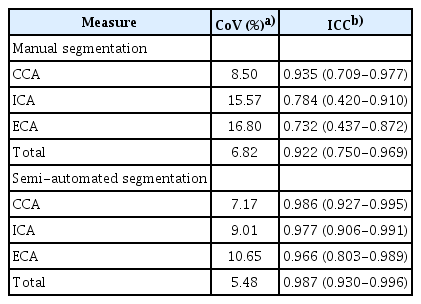
Inter-observer reliability of carotid vessel wall volume measurements on three-dimensional ultrasonography
Tables 4 and 5 summarize the intra-observer reliability, expressed as the CoV and ICC, respectively, between manual segmentation and semi-automated segmentation for carotid VWV measurements on 3DUS. From the perspective of carotid VWV measurements of individual carotid arteries, manual segmentation of the ICA (ICC, 0.756 to 0.878; 95% CI, 0.637 to 0.931; CoV, 18.51% to 22.82%) and ECA (ICC, 0.919 to 0.962; 95% CI, 0.868 to 0.979; CoV, 18.55% to 28.02%) had lower intra-observer reliability than that of the CCA (ICC, 0.934 to 0.966; 95% CI, 0.893 to 0.981; CoV, 14.99% to 17.62%). Semi-automated segmentation of the ICA (ICC, 0.958 to 0.978; 95% CI, 0.930 to 0.988; CoV, 29.54% to 34.04%) and ECA (ICC, 0.884 to 0.937; 95% CI, 0.815 to 0.965; CoV, 26.36% to 32.47%) also demonstrated lower intra-observer reliability than that of the CCA (ICC, 0.954 to 0.993; 95% CI, 0.924 to 0.996; CoV, 29.00% to 31.26%). Based on the total carotid VWV measurements, semi-automated segmentation (ICC, 0.968 to 0.989; 95% CI, 0.947 to 0.994; CoV, 22.90% to 24.77%) had higher intraobserver reliability than manual segmentation (ICC, 0.927 to 0.961; 95% CI, 0.882 to 0.979; CoV, 12.22% to 15.22%). The trend for higher ICC values in semi-automated segmentation than in manual segmentation was analogously obtained in individual carotid arteries, as reflected by the aforementioned intra-observer reliability values.
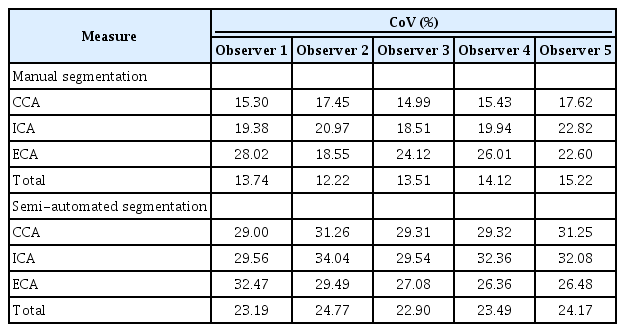
Intra-observer reliability expressed as the coefficient of variation for carotid vessel wall volume measurements on threedimensional ultrasonography
Discussion
Routine monitoring for asymptomatic carotid atherosclerotic plaque is essential for risk stratification and future ischemic stroke prediction [5-8]. It has been widely accepted that 3DUS and carotid VWV parameters outperform other conventional ultrasound techniques. To further facilitate the clinical utility of this advanced modality, the present study aimed to fill a research gap by contributing an extensive reliability analysis. This study compared the inter-observer and intra-observer reliability between manual segmentation and semi-automated segmentation on carotid VWV measurement on 3DUS. The study results demonstrated, (1) lower inter-observer and intra-observer reliability in carotid VWV measurements of the ICA and ECA than in measurements made using the CCA; (2) high inter-observer and intra-observer reliability for total carotid VWV measurements; and (3) higher inter-observer and intra-observer reliability with semi-automated segmentation than with manual segmentation.
These results demonstrated lower inter-observer and intra-observer reliability when measuring carotid VWV in the ICA and ECA than in measurements made using the CCA. This finding was seen for both manual and semi-automated segmentation. The measurements were maximally about 22% [(0.935-0.732)/0.935] less reproducible, which specifically occurred for the ECA against the CCA regarding inter-observer reliability in manual segmentation.
The relatively low reliability in the ICA and ECA regions may conceivably stem from the inherent anatomical properties of the segmentation targets. To a great extent, the ICA and ECA are situated at a less superficial location and run more deeply in the neck than the CCA [33]. The acoustic medium between the carotid arteries and the skin surface primarily consists of subcutaneous fat and muscle (i.e., platysma, sternothyroid, and sternocleidomastoid). When ultrasonography must penetrate a thicker fat-muscle layer during deep ICA and ECA imaging, the layer causes more interference and random scattering of the ultrasound beam, inducing more speckle noise contamination [34,35]. With a low signal-to-noise ratio, the contrast and edge strength of the media-adventitia and lumen-intima boundaries are eventually hindered [6]. Tracing the ICA and ECA branches could become more challenging and varied as a consequence of lower inter-observer and intra-observer reliability regardless of the type of segmentation. Meanwhile, to the best of the authors’ knowledge, no study has yet thoroughly compared the reliability of carotid VWV measurements across the CCA, ICA, and ECA. In light of the discovered sub-optimal reliability values, clinicians should be cautious about using 3DUS to measure carotid VWV for monitoring serial changes in the ICA and ECA.
In the present study, total carotid VWV measurement was supported, with excellent inter-observer and intra-observer reliability in both manual and semi-automated processing. Using the manual method, total carotid VWV measurements had an ICC of 0.922 (CoV, 6.82%) for inter-observer reliability and an ICC of 0.927-0.961 (CoV, 12.22% to 15.22%) for intra-observer reliability. The inter-observer results are comparable to the ICC of 0.91 reported by Egger et al. [21], and their reported intra-observer reliability (ICC, 0.95) also falls within the range of the current results. In comparison with another study conducted by Khan et al. [23], the present study had higher values for inter-observer reliability but compatible results for intra-observer reliability, as their team reported ICC values of 0.87 and 0.95 respectively. Regarding semi-automated segmentation, the total carotid VWV parameter in the current reliability analysis showed higher values than reported in the existing literature. Inter-observer and intra-observer reliability reached ICCs of 0.987 (CoV, 5.48%) and 0.968-0.989 (CoV, 22.90% to 24.77%) in this study. The previous maximum value was 0.90, reported by Khan et al. [23] for both inter-observer and intra-observer reliability.
Total carotid VWV measurements were highly reliable, likely because it requires observers to distinguish simply between the media-adventitia boundary and lumen-intima boundary. The shape is predominantly regular, low-ordered, and circular, making it more readily identifiable and straightforward for interpretation [22,23]. At the same time, the present results revealed modestly higher reliability than some prior findings. A major underlying reason relates to the methodological differences. The frame range for segmentation in the current study was kept controlled and unchanged in each repetition. This protocol is distinct from that used by Khan et al. [23], as re-defining frame notation was required amid every segmentation trial. In this case, additional variation in the carotid VWV outputs might be induced by the observers segmenting different frame ranges. This may explain the lower values reported for inter-observer and intra-observer segmentation reliability by Khan et al. [23], as compared to the present findings. Apart from this methodological discrepancy, the consideration of a mean rating particularly explains the higher inter-observer reliability of total carotid VWV measurements than previous reports. In essence, VWV was averaged across the five segmentation repetitions of each observer. While manipulating mean values, variations could be evened out by the extreme magnitudes within multiple trials [36]. As opposed to the single rating approach used by Khan et al. [23], the analysis of the mean ratings of total carotid VWV measurements eventually achieves better reliability than formerly reported.
This study found higher inter-observer and intra-observer reliability in semi-automated segmentation than in manual segmentation. For total carotid VWV, the semi-automated segmentation method showed better reproducibility between observers, with an ICC of 0.987 (95% CI, 0.930 to 0.996; CoV, 5.48%), than the manual method, with an ICC of 0.922 (95% CI, 0.750 to 0.969; CoV, 6.82%). This observation is in line with existing work on total carotid VWV, as a previous study showed higher inter-observer reliability using semi-automated segmentation (ICC, 0.90) than using manual segmentation (ICC, 0.87) [23]. Meanwhile, the total carotid VWV measurements in this study achieved superior intra-observer reliability (ICC, 0.968 to 0.989; 95% CI, 0.947 to 0.994; CoV, 22.90% to 24.77%) using semi-automated segmentation than using manual segmentation (ICC, 0.927 to 0.961; 95% CI, 0.882 to 0.979; CoV, 12.22% to 15.22%). The present findings also agree with other preliminary studies. Hossain et al. [6] reported higher intra-observer reliability in semi-automated segmentation (CoV, 5.2%) than in manual segmentation (CoV, 5.3%), although they only descriptively expressed the comparison in terms of relative variance. Khan et al. [23] similarly found a lower standard error of measurement in semi-automated processing (1.21%) than in manual processing (1.6%), and thereby concluded that this segmentation technique led to improved intra-observer reliability. Apart from total carotid VWV, individual arteries also showed improvements when segmented semi-automatically. The largest increase in reliability was approximately 32% [(0.966-0.732)/0.732], which took place for inter-observer reliability of the ECA. Thus, the current study suggests that semi-automated segmentation algorithms are robust and may perform more reliably than manual segmentation, in addition to the advantage demonstrated by previous studies that semi-automated programs shorten the post-processing duration [6,23,27-30].
In the vast majority of instances in this study, semi-automated segmentation was more reliable than manual segmentation. The reliability of ultrasonographic contouring is typically affected by the subjective and experience-dependent image perception of an individual observer. Hence, different observers may perceive the boundaries variably, implying an impact on the cursor placement during segmentation. As the semi-automated algorithm involves less operator intervention, it could detect boundaries and form contours in a more consistent, complex manner than manual outlining. If many 3DUS volume datasets are to be traced, it is likely to be difficult for observers to maintain visually reproducible manual recognition. Occasionally, carotid VWV measurement, for example at the ECA region, was performed reliably, with no large improvement from the semi-automated method in comparison to manual processing for repetitions within observers. The observed minor improvement is probably because of the image degradation in some 3DUS volume datasets, such as those acquired from obese individuals or participants with strong, muscular necks. A thicker fat-muscle layer causes more interference and random scattering of ultrasound beam than usual, resulting in more speckle noise interruption in these datasets [34,35]. In subjects with calcified plaques, the acoustic shadowing artifact sometimes also partially obscures the boundaries. As such, deviations could result not only from variation among observers, but also from variation in repeated attempts to recognize ill-defined boundaries. In addition to subject-related factors, Goldstein [37] commented that inconsistency could result from image pixelation effects in digital images. Spatial discretization during vessel boundary contouring may cause errors for both observers and semi-automated algorithms in this context. This, in turn, presumably gives rise to an abrupt change in the carotid VWV output to a similar extent in both segmentation methods.
One of the implicit limitations of this study is the memory bias and learning bias of observers, after interpreting the same sets of images over a long period of time. However, this factor unavoidably confounds any reliability analysis [32]. This study tried to diminish the error by re-randomizing the volume datasets, blinding observers, and setting a 24-hour interval between segmentation repetitions. In addition to measurement bias, scanning variability existed since observers only acquired each carotid volume once. The study omitted quantification of this variation, mainly because other investigators [21] have already examined this issue, which was beyond the intended scope of this study. However, several measures were adopted to minimize this source of error, including training observers with variability trials beforehand, randomly assigning observers to 3DUS volume acquisitions, and image quality supervision by an expert sonographer. Another pitfall of this study relates to the study population. Since the previous literature has been advocating using carotid VWV for asymptomatic plaque screening and future stroke prediction [5-8], the subject inclusion criteria of this study focused on healthy participants with no previous history of stroke, transient ischemic attack and cardiovascular disease. In other words, the acquired 3DUS volume data may or may not have contained carotid atherosclerotic plaques due to the study’s asymptomatic screening nature. The findings of this study may therefore have limited generalizability to carotid VWV measurements of arteries with definitive atherosclerotic plaques. For this reason, information on the stenosis percentage is shown in Table 2. Apart from the limitation of recruiting a healthy population, it was not possible to include volume datasets with highly situated carotid bifurcations in the study subject pool. The ICA and ECA regions of these subjects were extensively obscured by the bony mandible, implying an inferior visualization. This acquisition constraint was unable to be resolved since the volume ultrasound transducer has a bulky footprint. Flexibility in angulation is greatly inhibited at the mid-cervical region. The present analysis eventually excluded the related volume datasets during the acquisition stage. Likewise, future work is needed to examine precautions that should be taken in cases of highly situated carotid bifurcations or other anatomical variations.
In summary, this study found higher inter-observer and intra-observer reliability in carotid VWV measurements using the CCA than those obtained using the ICA and ECA, high inter-observer and intra-observer reliability in total carotid VWV measurements, and higher inter-observer and intra-observer reliability with semi-automated segmentation than with manual segmentation. This study contributes to the development of carotid VWV measurement in 3DUS by reaffirming the high reliability of total carotid VWV measurements, in agreement with the existing literature; and validating semi-automated segmentation as being efficient without compromising reliability.
Notes
Author Contributions
Conceptualization: Chan CW, Chow SCC, Ying M. Data acquisition: Chan CW, Chow SCC, Kwok MH, Ngan KCT, Or TH, Gunda ST. Data analysis or interpretation: Chan CW, Chow SCC, Kwok MH, Ngan KCT, Or TH. Drafting of the manuscript: Chan CW, Chow SCC, Kwok MH, Ngan KCT, Or TH, Gunda ST. Critical revision of the manuscript: Ying M. Approval of the final version of the manuscript: all authors.
No potential conflict of interest relevant to this article was reported.
Acknowledgements
The project was supported by research funding from the Hong Kong Polytechnic University (Project ID: P0035203).
References
Article information Continued
Notes
Key point
Clinicians should be alerted regarding the relatively low reliability of internal carotid artery and external carotid artery vessel wall volume (VWV) measurements. The high reliability of total carotid VWV was reaffirmed, in accordance with the previous literature. Efficient semi-automated segmentation should be considered in three-dimensional ultrasound carotid VWV measurements, since it does not compromise reliability.